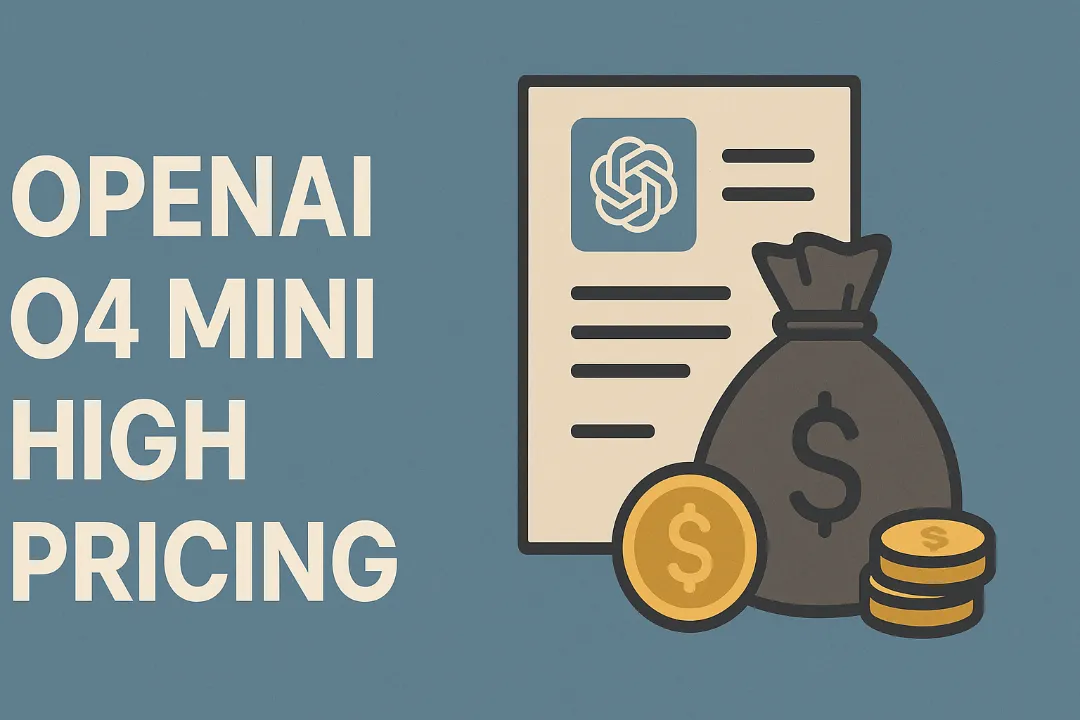
GPT O4 Mini High Pricing: Complete Guide to OpenAI's Latest Model (2025)
Openai O4 Mini High Pricing: Complete Guide to OpenAI's Latest Model (2025)
On April 16, 2025, OpenAI expanded its O-series with the introduction of the O4-mini-high model, adding to its suite of reasoning-focused AI systems. This new offering represents OpenAI's commitment to providing more cost-effective solutions without sacrificing significant performance. As organizations and developers seek to optimize their AI expenditures, understanding the pricing, capabilities, and potential use cases of O4-mini-high has become essential.
In this comprehensive guide, we'll examine the O4-mini-high model in detail, analyzing its pricing structure relative to other OpenAI models, exploring its technical specifications, reviewing its performance on benchmark tests, and providing insights into its most suitable applications. Whether you're an AI engineer, product manager, or business leader, this analysis will help you determine if O4-mini-high offers the right balance of cost and capabilities for your AI strategy.
What is O4-mini-high?
O4-mini-high is OpenAI's latest small reasoning model in their o-series, designed to provide exceptional reasoning performance while maintaining cost efficiency. It follows the company's "mini" naming convention, indicating a smaller, more affordable version of their full-sized O4 model (which is still in development).
Key Features at a Glance
- Model Type: Small reasoning model in the O-series
- Release Date: April 16, 2025
- Knowledge Cutoff: May 31, 2024
- Context Window: 200,000 tokens
- Maximum Output: 100,000 tokens
- Multimodal Capabilities: Text and image input, text output
- Optimized For: Fast, effective reasoning with exceptional performance in coding and visual tasks
O4-mini-high represents an evolution in OpenAI's reasoning model lineup, providing significant improvements over the earlier O3-mini-high while maintaining a similar pricing structure. The model excels particularly in STEM-related tasks, making it an attractive option for technical use cases that require robust reasoning capabilities.
O4-mini-high Pricing Structure
One of the most compelling aspects of O4-mini-high is its pricing, which offers significant cost advantages compared to larger models like GPT-4o while delivering impressive performance.
Token-Based Pricing
Usage Type | Cost (per million tokens) |
---|---|
Input | $1.10 |
Cached Input | $0.275 |
Output | $4.40 |
This pricing structure makes O4-mini-high approximately 2.3 times cheaper than GPT-4o, which costs $2.50 per million tokens for input and $10.00 per million tokens for output.
Pricing Comparison with Other OpenAI Models
To better understand O4-mini-high's position in OpenAI's pricing hierarchy, let's compare it with other models in the company's lineup:
Model | Input (per million tokens) | Output (per million tokens) | Cost Ratio (vs. O4-mini-high) |
---|---|---|---|
O4-mini-high | $1.10 | $4.40 | 1.0x |
GPT-4o | $2.50 | $10.00 | ~2.3x |
O3-mini-high | $1.10 | $4.40 | 1.0x |
O1 Preview | $15.00 | $60.00 | ~13.6x |
O1 | $15.00 | $60.00 | ~13.6x |
O1-mini | $1.10 | $4.40 | 1.0x |
As shown in the table, O4-mini-high maintains the same pricing structure as O3-mini-high and O1-mini, making it an economical choice in OpenAI's model lineup. This pricing consistency across mini models demonstrates OpenAI's commitment to providing cost-effective AI solutions.
Technical Specifications and Capabilities
O4-mini-high comes with impressive technical specifications that enable it to handle complex tasks with efficiency and accuracy.
Context Window and Output Size
The model features a 200,000 token context window and can generate up to 100,000 tokens in a single response. This extensive context window—larger than GPT-4o's 128K tokens—allows O4-mini-high to process and reason over lengthy documents, conversations, or code bases in a single prompt.
Reasoning Capabilities
O4-mini-high's most notable feature is its enhanced reasoning ability. The model excels at:
- Mathematical Problem Solving: Demonstrated by high performance on benchmarks like AIME and MATH
- Scientific Reasoning: Strong results on the GPQA (Graduate-level Physics Questions Assessment)
- Code Generation and Modification: Impressive results on software engineering benchmarks
- Visual Reasoning: Enhanced capabilities for understanding and reasoning with images
Supported Features
O4-mini-high supports a range of developer features, including:
- Function calling
- Streaming
- JSON mode
- Seed parameter
- Developer messages
- Reasoning tokens (for enhanced problem-solving)
- Multiple reasoning effort settings (low, medium, high)
Performance Benchmarks
The true value of an AI model is revealed through its performance on standardized benchmarks. O4-mini-high demonstrates impressive capabilities across various testing frameworks.
Aider Polyglot Benchmark
According to the Aider leaderboard, O4-mini-high achieves a 72.0% success rate on the polyglot benchmark, which tests performance across multiple programming languages (C++, Go, Java, JavaScript, Python, and Rust). This places it third in the rankings, behind O3 (high) at 79.6% and Gemini 2.5 Pro Preview at 72.9%.
Mathematical and Scientific Benchmarks
O4-mini-high shows exceptional performance on mathematical and scientific reasoning benchmarks:
Benchmark | O4-mini-high Score | Comparison to Other Models |
---|---|---|
AIME2024 | 93.4% (no tools) | Significantly outperforms GPT-4o (13.1%) |
AIME2025 | 92.7% (no tools) | N/A for most other models |
GPQA | 81.4% (no tools, high effort) | Outperforms GPT-4o (46%) |
MathVista | 84.3% (Visual Math Reasoning) | Outperforms GPT-4o (61.4%) |
SWE-Bench | 68.1% (Verified) | Outperforms GPT-4o (33.2%) |
MMMU | 81.6% | Outperforms GPT-4o (68.7%) |
These benchmark results highlight O4-mini-high's exceptional reasoning capabilities, particularly in STEM domains. The model consistently outperforms larger models like GPT-4o on specialized reasoning tasks while maintaining a much lower price point.
User Experiences and Feedback
As a recently released model, comprehensive user feedback on O4-mini-high is still emerging. However, initial reactions from early adopters highlight several key aspects of the model's real-world performance.
Developer Impressions
"O4-mini-high has revolutionized our code refactoring workflow. We're seeing about 70% reduction in time spent on routine code modifications, and the cost is significantly lower than what we were paying with GPT-4o. The reasoning capabilities are impressive for a model at this price point." - Alex M., Software Engineering Lead
"The balance between cost and performance is what makes O4-mini-high stand out. For our data analysis tasks, it provides nearly the same quality as O3 at a fraction of the cost. We've been able to scale our AI operations significantly thanks to the efficiency of this model." - Priya K., Data Science Director
"While O4-mini-high excels at technical reasoning, we've found it slightly less nuanced in creative writing tasks compared to GPT-4o. However, for our primary use cases in technical documentation and code generation, it's become our go-to model." - Marco T., Technical Documentation Manager
Comparative Strengths and Limitations
Based on user feedback and benchmark results, here's a summary of O4-mini-high's strengths and limitations:
Strengths:
- Exceptional performance on mathematical and scientific reasoning tasks
- Strong coding capabilities across multiple programming languages
- Excellent cost-to-performance ratio
- Large context window (200K tokens)
- Ability to process and reason with images
Limitations:
- Less creative versatility compared to GPT-4o
- Occasional inconsistencies in complex multi-step reasoning
- Limited deployment options compared to more established models
- Less robust tool-use capabilities compared to full-sized reasoning models
Use Cases: Where O4-mini-high Excels
O4-mini-high's combination of strong reasoning capabilities and cost efficiency makes it particularly well-suited for specific applications.
Ideal Applications
Software Development and Code Review
- Automated code generation and refactoring
- Debugging and optimization
- Technical documentation generation
- Code review and quality assurance
Education and Research
- Mathematical problem solving
- Scientific paper analysis
- Educational content generation
- Research assistance and literature review
Data Analysis
- Pattern identification in large datasets
- Statistical analysis interpretation
- Data visualization planning
- Technical report generation
Technical Support
- Troubleshooting technical issues
- Creating step-by-step guides
- Answering technical questions
- Analyzing error logs and providing solutions
Sample Application: Automated Code Refactoring
An example workflow using O4-mini-high for code refactoring might look like this:
- Developer uploads legacy codebase for analysis
- O4-mini-high identifies outdated patterns, security vulnerabilities, and optimization opportunities
- Model generates refactoring suggestions with code examples
- Developer reviews and implements suggestions
- O4-mini-high validates changes and suggests additional improvements
This workflow leverages O4-mini-high's strong reasoning and coding capabilities while benefiting from its cost efficiency, allowing teams to process larger codebases at a lower cost compared to using models like GPT-4o or O1.
O4-mini-high vs. Competitors: Detailed Comparison
To provide a more comprehensive understanding of O4-mini-high's position in the market, let's compare it with key competitors across several dimensions.
OpenAI Models Comparison
Feature | O4-mini-high | O3-mini-high | GPT-4o | O1 |
---|---|---|---|---|
Input Price (per million tokens) | $1.10 | $1.10 | $2.50 | $15.00 |
Output Price (per million tokens) | $4.40 | $4.40 | $10.00 | $60.00 |
Context Window | 200K | 200K | 128K | 128K |
Max Output Tokens | 100K | 100K | 16.4K | 32.8K |
Knowledge Cutoff | May 31, 2024 | Unknown | October 2023 | October 2023 |
Release Date | April 16, 2025 | January 31, 2025 | August 6, 2024 | December 2024 |
Polyglot Benchmark Score | 72.0% | 60.4% | 23.1% | 61.7% |
Multimodal Capabilities | Text + Image | Text | Text + Image + Audio | Text |
Tool Use Support | Basic | Basic | Advanced | Advanced |
Competitive Position
The comparison shows that O4-mini-high offers several advantages:
- Price Efficiency: Maintains the same pricing as O3-mini-high but with improved performance
- Context Size: Offers a larger context window than GPT-4o and O1
- Benchmark Performance: Delivers superior reasoning performance compared to its price tier
- Knowledge Recency: Contains more recent knowledge compared to GPT-4o and O1
These advantages position O4-mini-high as an excellent choice for organizations seeking strong reasoning capabilities without the premium price tag of O1 or the full-sized O3/O4 models.
Implementation Strategies
For organizations considering implementing O4-mini-high, here are some strategic recommendations to maximize value and effectiveness.
Best Practices
Optimize for Reasoning Tasks: Direct complex reasoning, mathematical, and coding tasks to O4-mini-high to leverage its strengths.
Implement a Hybrid Model Approach: Use O4-mini-high for reasoning-heavy workloads while reserving models like GPT-4o for creative or multimodal tasks that require greater flexibility.
Utilize Reasoning Effort Settings: Experiment with the three reasoning effort settings (low, medium, high) to find the optimal balance between performance and cost for your specific use cases.
Leverage the Large Context Window: Take advantage of O4-mini-high's 200K token context window for tasks involving large documents or codebases.
Structure Prompts for Reasoning: Design prompts that break complex problems into step-by-step reasoning processes to maximize O4-mini-high's problem-solving capabilities.
Sample Integration Approaches
API Integration Example
import openai
client = openai.OpenAI(api_key="your-api-key")
response = client.chat.completions.create(
model="o4-mini",
messages=[
{"role": "system", "content": "You are a helpful assistant specialized in solving complex mathematical problems. Show your reasoning step by step."},
{"role": "user", "content": "Find all the real solutions to the equation x^3 - 6x^2 + 11x - 6 = 0."}
],
reasoning_effort="high" # Options: low, medium, high
)
print(response.choices[0].message.content)
This example demonstrates how to leverage O4-mini-high's reasoning capabilities by setting the reasoning effort to "high" for complex mathematical problem solving.
The Future of O4-mini-high
As O4-mini-high is a recent release, its capabilities and applications will likely evolve over time. Here are some potential developments to watch for:
Anticipated Improvements
Enhanced Tool Integration: Future updates may include improved integration with external tools and services.
Specialized Fine-tuning Options: OpenAI may introduce domain-specific fine-tuning for O4-mini-high to enhance performance in particular industries.
Extended Multimodal Capabilities: Additional image understanding and processing capabilities may be added to strengthen O4-mini-high's multimodal reasoning.
Community-Driven Use Cases: As more developers adopt the model, expect to see innovative applications and optimizations emerge from the community.
The Evolving O-Series Landscape
O4-mini-high represents just one component of OpenAI's evolving O-series strategy. If past patterns hold, we might anticipate:
- A full-sized O4 model with expanded capabilities
- Potential specialized versions targeting specific domains
- Further integrations with OpenAI's broader ecosystem
- Continued pricing optimizations to reflect market dynamics
For organizations investing in AI infrastructure, understanding these potential trajectories can help inform long-term strategic planning.
Conclusion: Is O4-mini-high Right for You?
O4-mini-high represents a significant advancement in cost-effective AI reasoning capabilities. Its impressive performance across technical benchmarks, combined with its economical pricing, makes it an attractive option for organizations looking to deploy advanced AI capabilities at scale.
Ideal For:
- Technical organizations with reasoning-heavy workloads
- Software development teams seeking cost-effective coding assistance
- Educational institutions requiring mathematical and scientific reasoning
- Organizations with budget constraints but high performance requirements
- Projects requiring large context windows and extended outputs
Less Suitable For:
- Applications requiring advanced creative content generation
- Complex multimodal tasks beyond image understanding
- Use cases dependent on extensive tool use
- Applications requiring the absolute cutting-edge in reasoning capabilities
The introduction of O4-mini-high continues OpenAI's trend of making advanced AI capabilities more accessible through strategic model sizing and pricing. For many organizations, it represents the sweet spot in the price-performance curve, offering capabilities that were previously available only at premium price points.
As with any AI technology, the true value of O4-mini-high will be determined by how effectively it can be integrated into existing workflows and systems. Organizations that take the time to understand its capabilities and limitations will be best positioned to leverage its strengths for competitive advantage.
FAQs About O4-mini-high Pricing and Features
General Questions
Q: When was O4-mini-high released? A: O4-mini-high was released on April 16, 2025.
Q: How does O4-mini-high compare to O3-mini-high? A: O4-mini-high maintains the same pricing structure as O3-mini-high but delivers improved performance across reasoning benchmarks, particularly in STEM domains.
Q: Can O4-mini-high process images? A: Yes, O4-mini-high can process both text and image inputs while generating text outputs.
Pricing and Usage
Q: How much does O4-mini-high cost? A: O4-mini-high costs $1.10 per million tokens for input and $4.40 per million tokens for output.
Q: Is O4-mini-high available through the OpenAI API? A: Yes, O4-mini-high is accessible through the OpenAI API.
Q: Are there any rate limits for O4-mini-high? A: Yes, there are rate limits, though specific limits depend on your usage tier with OpenAI.
Technical Capabilities
Q: What is the context window size for O4-mini-high? A: O4-mini-high features a 200,000 token context window.
Q: Does O4-mini-high support function calling? A: Yes, O4-mini-high supports function calling, streaming, JSON mode, and other developer features.
Q: What programming languages does O4-mini-high perform well with? A: Based on benchmark testing, O4-mini-high performs well across multiple languages including Python, JavaScript, Java, C++, Go, and Rust.
This comprehensive analysis should help you determine whether O4-mini-high is the right choice for your AI implementation needs, balancing cost considerations with performance requirements.